Tech notes
Localizing Ground-Penetrating Radar
Innovative ground-penetrating radar enables autonomous vehicle lane keeping in snow, rain, and fog. The technology could reduce navigational failure rates by orders of magnitude, even in fair conditions.
Autonomous vehicles aim to prevent most of the 35,000 deaths and 2.3 million injuries caused annually by motor vehicle accidents in the United States. While significant progress has been made toward commercial autonomous vehicles, current systems do not operate reliably in adverse conditions, such as snow, fog, and heavy rain. Even in fair-weather conditions, autonomous vehicle disengagement rates (i.e., the number of times a human has to retake control of the vehicle) are orders of magnitude higher than they will need to be for safe vehicle operation.
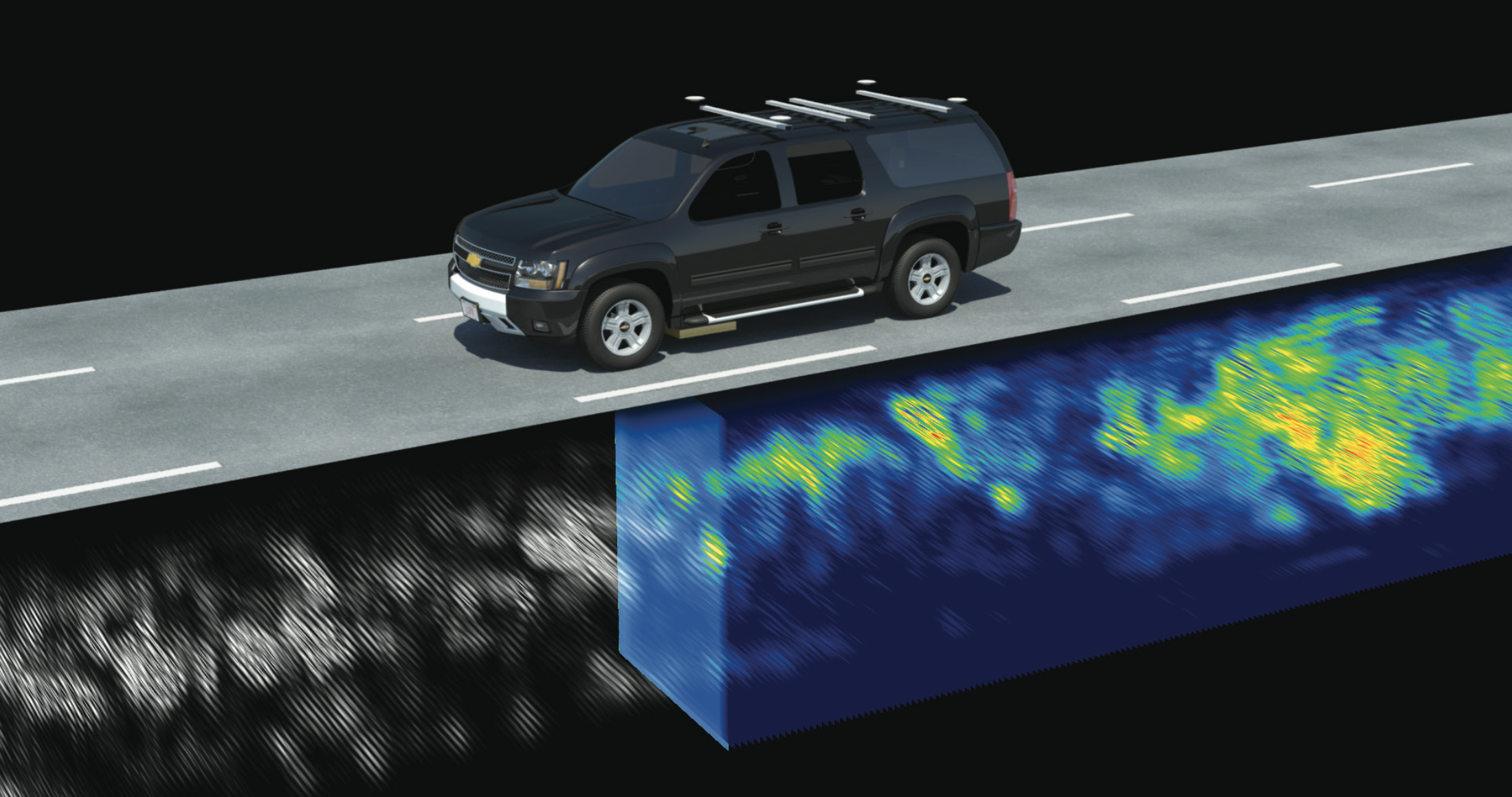
MIT Lincoln Laboratory has developed a sensor that provides real-time position estimates in challenging weather and road conditions and that is independent of current localization modes. Localizing Ground-Penetrating Radar (LGPR) uses very-high-frequency (VHF) radar reflections of underground features to generate baseline maps and then matches current GPR reflections to those maps to estimate a vehicle's location. LGPR uses relatively deep subsurface features as points of reference because these features are inherently stable and less susceptible to erosion or damage over time. It utilizes VHF radio waves because they can penetrate rain, fog, dust, and snow. Because all sensors can fail, the best way to achieve robustness is to fuse several independent approaches so no one failure mode causes a significant lane-keeping error.
LGPR Methodology
For subsurface sensing, GPR is one of the most versatile and prolific sensing modalities today. GPR systems work by sending a pulse of electromagnetic radiation into the ground and measuring reflections that originate from scattering points below the surface. Reflections occur at the interface between objects that have different electromagnetic properties, such as pipes, roots, and rocks in the surrounding "dirt." However, it is not these discrete objects but rather the natural inhomogeneity in subterranean geology that often dominates GPR reflection profiles. Soil layers and variations in moisture content cause reflections in the data. Thus, GPR paints a fairly complete picture of the subsurface environment.
With few exceptions, nearly every discrete object and soil feature is captured, provided that it is not significantly smaller than a wavelength and that it has sufficient contrast with the surrounding soil. The premise of GPR localization is that these subsurface features are sufficiently unique and static to permit their use as identification of the precise location at which their reflections were collected.
Mapping
The first step in the LGPR process is to develop a map of the environment below the road. The GPR data of subterranean "objects" are simply collected along with GPS tags to form the initial database of subsurface features. This subsurface map is then used as a reference dataset to estimate vehicle location on subsequent visits. Current work has demonstrated the temporal stability of LGPR maps through six-month and one-year time periods.
Tracking
Next, online localization is performed in several steps. When the vehicle is in motion, data regions are periodically fetched from the database for matching. A search region around the initial location estimate is populated with "particles" representing candidate locations and orientations. An algorithm iteratively evaluates the particles to narrow the search for the maximum correlation within the vehicle's five-dimensional space (easting, northing, height, roll, and heading). After several iterations, the highest correlation particle is chosen and its position used.
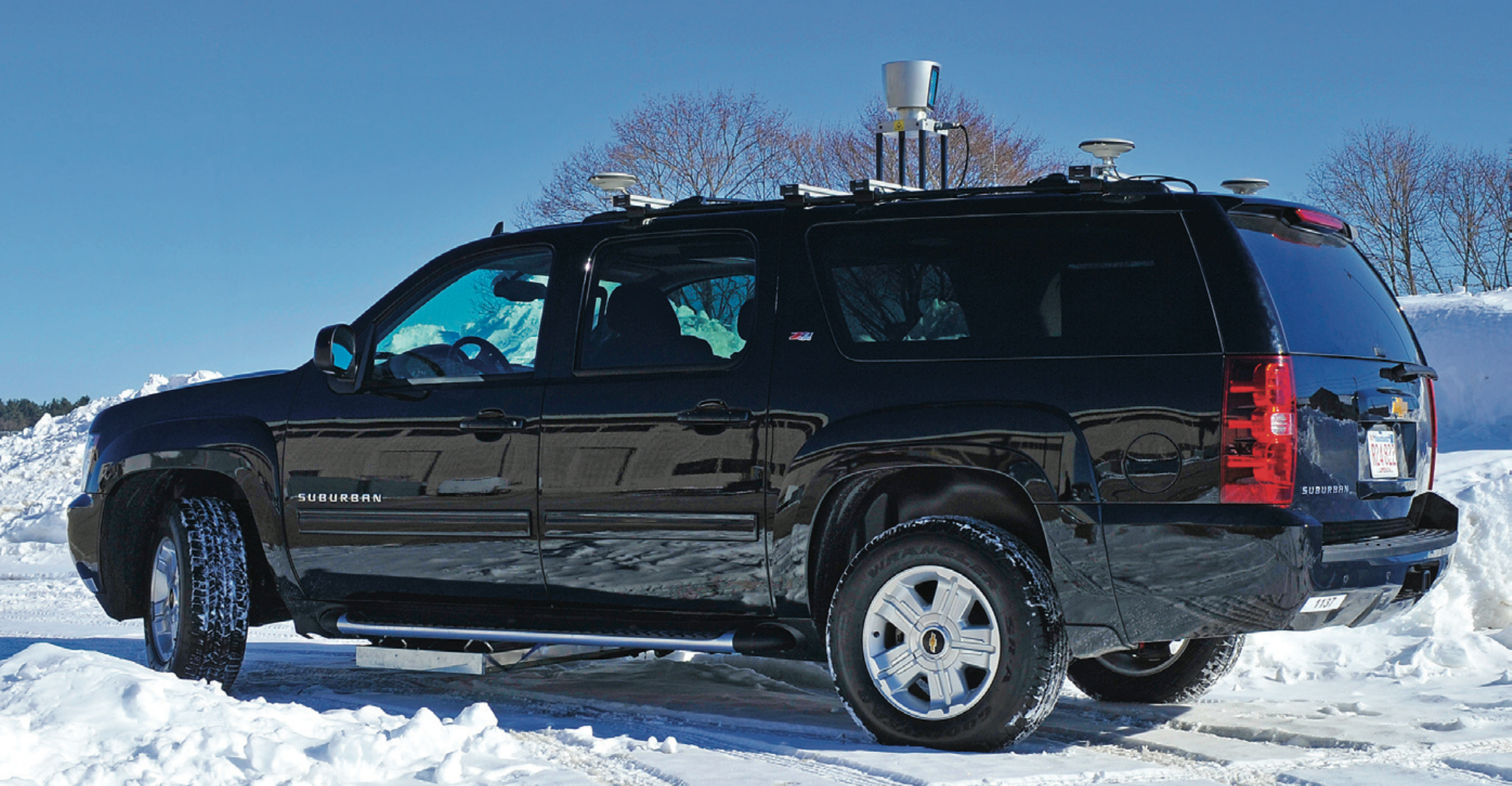
LGPR Design
The basic component of LGPR is a unique waterproof 12-element antenna array that uses a custom VHF stepped-frequency continuous-wave radar. The VHF system penetrates deeper than typical GPR systems to capture deeper, more stable geological features. Also, because VHF frequencies are inherently insensitive to small objects (e.g., a small soda can on the surface will be ignored because of its small VHF radar cross section), their use ensures that new reflections are from the types of geological features cataloged in the baseline data.
Several key modifications to traditional GPR were fundamental to the design:
- The spacing between array elements is approximately one-tenth of a center-frequency wavelength. This resolution is finer than typically seen in GPR arrays and enables high-fidelity matching to baseline data.
- All elements in the array have identical near-field patterns. This requirement allows path retraversal to resolve pass-to-pass offset or misalignment.
- A simple design allows for future mass production costs below $300 per sensor.
Demonstrated Capability
The LGPR system was tested over 1000s of miles on paved and unpaved roads in four U.S. states and was used by the U.S. military to navigate multiple vehicles over more than 1000 miles in Afghanistan's demanding environments. The LGPR method showed robust performance in these trials.
The article titled "Localizing Ground Penetrating RADAR: A Step Toward Robust Autonomous Ground Vehicle Localization," published in the January 2016 issue of the Journal of Field Robotics, describes Lincoln Laboratory's demonstration of 4 cm cross-track localization achieved by a vehicle driven at 60 mph under fair weather conditions. Recent work demonstrated real-time centimeter-level, highway-speed, nighttime localization during a snowstorm that had obscured all lane markings.
Recent research, presented at the Autonomous Vehicle Symposium 2017, has shown that the LGPR maps are stable over six-months and longer time periods, thereby reducing map upkeep requirements.
Benefits of LGPR
The main advantages of adding the LGPR to an autonomous vehicle sensor suite are its independence and its ability to operate under conditions that incapacitate other localization sensors, such as optical systems. Because the LGPR deduces location on the basis of stable underground features, it can provide position estimates even if it encounters severe weather, unpaved roads and faded markings, altered roads, dynamic environments, or GPS-denied areas. Fusing LGPR with lidar or other remote sensing methods provides improved localization capabilities for autonomous vehicles.
Furthermore, maps from below-road features that the LGPR captures can be useful for infrastructure inspection, such as finding underground sinkholes or detecting structural weaknesses in bridges. Because the LGPR compares the data it collects against prior scans of the terrain, changes in the scans (e.g., subsurface deterioration) can be readily detected.
Posted July 2017
top of page